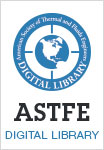
ISSN Online: 2379-1748
7th Thermal and Fluids Engineering Conference (TFEC)
SJR:
0.152
SNIP:
0.14
CiteScore™::
0.5
Indexed in
APPLYING AN ARTIFICIAL NEURAL NETWORK TO MODEL INTUBE HEAT TRANSFER IN LAMINAR FLOW
Abstract
The calculation of heat transfer to or from fluid flowing in a pipe or tube is common in chemical and process plant design. Both forced and free convection, separately or in combination as mixed convection, affect heat transfer. Graphical regime maps characterize the flow using the length-to-diameter ratio, as well as Grashof, Prandtl, and Reynolds numbers. Additionally, the average Nusselt number for laminar flows depends on the relative development lengths of the velocity and thermal boundary layers, the thermal boundary condition (uniform wall temperature or uniform heat flux), the pipe orientation, and the bulk-to-wall viscosity ratio. In our study, we used open literature data and correlation for horizontal tubes to form data tuples, the majority of which were used for training and the rest for testing. Scaled data were fit with an artificial neural network (ANN) using a multilayer perceptron with a single hidden layer. We determined that the network can predict even noisy data with less error
then literature correlations which are understandably tuned to the particular underlying data. We describe the usefulness and shortcomings of the ANN method in complementing traditional analysis of complex thermal engineering phenomena.
KEY WORDS:
laminar intube flow, artificial neural network