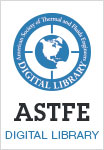
ISSN Online: 2379-1748
8th Thermal and Fluids Engineering Conference (TFEC)
XGBOOST-BASED MODEL FOR PREDICTION OF HEAT TRANSFER COEFFICIENTS IN LIQUID COLD PLATES
Resumo
Extreme gradient boosting (XGBoost) algorithm is a newly developed machine learning (ML) technique with demonstrated excellent accuracy and performance in modelling complex processes in science and engineering. In the present study, an XGBoost-based model is developed to predict heat transfer coefficients in liquid cold plates (CPs) subjected to surface roughness. The CPs operate in turbulent flow over a wide range of Reynolds numbers. Roughness sizes range from zero (smooth surface) to 0.5 mm. The input dataset for training the XGBoost model is prepared using a computational fluid dynamics (CFD) approach and by solving three-dimensional fluid flow and heat transfer inside the CPs. It was found that the model exhibits excellent accuracy such that 63% and 90% of heat transfer coefficients are predicted within ±10% and ±20% of true values, respectively. The present finding suggests XGBoost as an effective modelling tool for performance analysis of thermal management solutions, specifically when there is limited performance data available in literature.
Palavras-chave:
Machine learning, XGBoost, Heat transfer coefficient prediction, Surface roughness, CFD.